When you see the legendary ouroboros, it’s completely logical to assume “well, that won’t last.” A potent image, swallowing your individual tail — however tough in apply. It stands out as the case for AI as effectively, which in accordance with a brand new research, could also be prone to “model collapse” after just a few rounds of being educated on information it generated itself.
In a paper revealed in Nature, British and Canadian researchers led by Ilia Shumailov at Oxford present that at this time’s machine studying fashions are basically weak to a syndrome they name “model collapse.” As they write within the paper’s introduction:
We uncover that indiscriminately studying from information produced by different fashions causes “model collapse” — a degenerative course of whereby, over time, fashions neglect the true underlying information distribution …
How does this occur, and why? The course of is definitely fairly straightforward to know.
AI fashions are pattern-matching programs at coronary heart: They study patterns of their coaching information, then match prompts to these patterns, filling within the most probably subsequent dots on the road. Whether you ask “what’s a good snickerdoodle recipe?” or “list the U.S. presidents in order of age at inauguration,” the mannequin is mainly simply returning the most probably continuation of that sequence of phrases. (It’s completely different for picture turbines, however related in some ways.)
But the factor is, fashions gravitate towards the commonest output. It gained’t offer you a controversial snickerdoodle recipe however the most well-liked, unusual one. And in the event you ask a picture generator to make an image of a canine, it gained’t offer you a uncommon breed it solely noticed two photos of in its coaching information; you’ll most likely get a golden retriever or a Lab.
Now, mix these two issues with the truth that the online is being overrun by AI-generated content material, and that new AI fashions are prone to be ingesting and coaching on that content material. That means they’re going to see a lot of goldens!
And as soon as they’ve educated on this proliferation of goldens (or middle-of-the highway blogspam, or pretend faces, or generated songs), that’s their new floor fact. They will assume that 90% of canine actually are goldens, and subsequently when requested to generate a canine, they are going to increase the proportion of goldens even larger — till they mainly have misplaced observe of what canine are in any respect.
This great illustration from Nature’s accompanying commentary article exhibits the method visually:
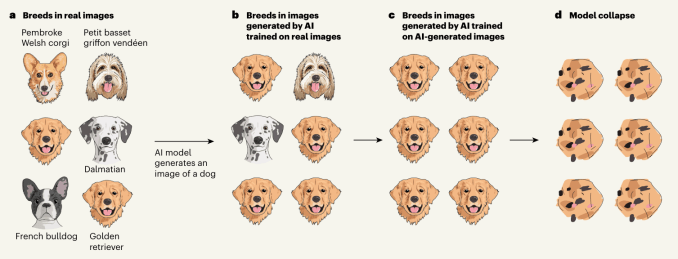
An analogous factor occurs with language fashions and others that, basically, favor the commonest information of their coaching set for solutions — which, to be clear, is normally the suitable factor to do. It’s probably not an issue till it meets up with the ocean of chum that’s the public net proper now.
Basically, if the fashions proceed consuming one another’s information, maybe with out even realizing it, they’ll progressively get weirder and dumber till they collapse. The researchers present quite a few examples and mitigation strategies, however they go as far as to name mannequin collapse “inevitable,” a minimum of in concept.
Though it might not play out because the experiments they ran present it, the chance ought to scare anybody within the AI area. Diversity and depth of coaching information is more and more thought of the one most necessary issue within the high quality of a mannequin. If you run out of information, however producing extra dangers mannequin collapse, does that basically restrict at this time’s AI? If it does start to occur, how will we all know? And is there something we will do to forestall or mitigate the issue?
The reply to the final query a minimum of might be sure, though that ought to not alleviate our considerations.
Qualitative and quantitative benchmarks of information sourcing and selection would assist, however we’re removed from standardizing these. Watermarks of AI-generated information would assist different AIs keep away from it, however to date nobody has discovered an appropriate technique to mark imagery that means (effectively … I did).
In reality, firms could also be disincentivized from…