One of the promoting factors of Google’s flagship generative AI fashions, Gemini 1.5 Pro and 1.5 Flash, is the quantity of information they’ll supposedly course of and analyze. In press briefings and demos, Google has repeatedly claimed that the fashions can accomplish beforehand unimaginable duties because of their “long context,” like summarizing a number of hundred-page paperwork or looking throughout scenes in movie footage.
But new analysis means that the fashions aren’t, in truth, superb at these issues.
Two separate research investigated how nicely Google’s Gemini fashions and others make sense out of an infinite quantity of information — assume “War and Peace”-length works. Both discover that Gemini 1.5 Pro and 1.5 Flash wrestle to reply questions on massive datasets appropriately; in a single collection of document-based exams, the fashions gave the appropriate reply solely 40% 50% of the time.
“While models like Gemini 1.5 Pro can technically process long contexts, we have seen many cases indicating that the models don’t actually ‘understand’ the content,” Marzena Karpinska, a postdoc at UMass Amherst and a co-author on one of many research, instructed TechCrunch.
Gemini’s context window is missing
A mannequin’s context, or context window, refers to enter knowledge (e.g., textual content) that the mannequin considers earlier than producing output (e.g., further textual content). A easy query — “Who won the 2020 U.S. presidential election?” — can function context, as can a film script, present or audio clip. And as context home windows develop, so does the dimensions of the paperwork being match into them.
The latest variations of Gemini can absorb upward of two million tokens as context. (“Tokens” are subdivided bits of uncooked knowledge, just like the syllables “fan,” “tas” and “tic” within the phrase “fantastic.”) That’s equal to roughly 1.four million phrases, two hours of video or 22 hours of audio — the biggest context of any commercially accessible mannequin.
In a briefing earlier this yr, Google confirmed a number of pre-recorded demos meant as an instance the potential of Gemini’s long-context capabilities. One had Gemini 1.5 Pro search the transcript of the Apollo 11 moon touchdown telecast — round 402 pages — for quotes containing jokes, after which discover a scene within the telecast that appeared just like a pencil sketch.
VP of analysis at Google DeepMind Oriol Vinyals, who led the briefing, described the mannequin as “magical.”
“[1.5 Pro] performs these sorts of reasoning tasks across every single page, every single word,” he stated.
That may need been an exaggeration.
In one of many aforementioned research benchmarking these capabilities, Karpinska, together with researchers from the Allen Institute for AI and Princeton, requested the fashions to guage true/false statements about fiction books written in English. The researchers selected latest works in order that the fashions couldn’t “cheat” by counting on foreknowledge, and so they peppered the statements with references to particular particulars and plot factors that’d be unimaginable to understand with out studying the books of their entirety.
Given an announcement like “By using her skills as an Apoth, Nusis is able to reverse engineer the type of portal opened by the reagents key found in Rona’s wooden chest,” Gemini 1.5 Pro and 1.5 Flash — having ingested the related ebook — needed to say whether or not the assertion was true or false and clarify their reasoning.
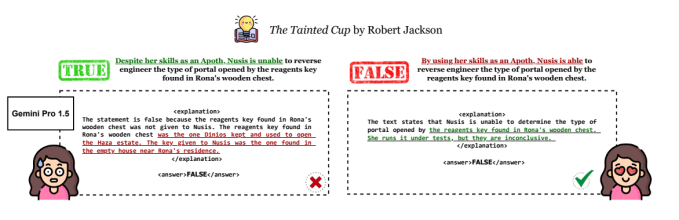
Tested on one ebook round 260,000 phrases (~520 pages) in size, the researchers discovered that 1.5 Pro answered the true/false statements appropriately 46.7% of the time whereas Flash answered appropriately solely 20% of the time. That means a coin is considerably higher at answering questions in regards to the ebook than Google’s newest machine studying mannequin. Averaging all of the benchmark outcomes, neither mannequin managed to realize larger than random likelihood when it comes to question-answering accuracy.
“We’ve seen that the fashions have…